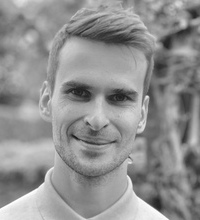
Research Scientist at NNAISENSE
Laboratory of Intelligent Decision Support Systems
Institute of Computing Science, PUT
pawel
Lugano, Switzerland
Hi! I'm Paweł. I'm a passionate computer scientist working in the field of machine learning. I focus on building intelligent solutions to complex industry problems where conventional engineering methods are often not powerful enough to provide satisfactory results. Neural networks and deep learning are at the heart of my interests. I particularly enjoy combining various bio-inspired learning paradigms into powerful hybrid learning algorithms.
Research
At the very core, my research concerns the design of efficient algorithms that automatically improve with experience. In recent years, I have developed many such algorithms across different fields, including computer vision, reinforcement learning, and evolutionary computation.
Some of my achievements include devising advanced methods for object detection and segmentation in 2D and 3D medical images, training game-playing agents that achieve super-human performance through the principle of reinforcement learning, or automatically synthesizing computer programs from a specification using hybrid evolutionary algorithms.
Much of my research to date has involved the exploration of various approaches to computational intelligence with an emphasis on bio-inspired methods such as neural networks and neuroevolution. A common theme that emerges in my work is providing learning algorithms with the capability to collect and exploit rich information on their internal learning process, aiming to improve convergence properties and overall performance.
Most recently, I've shifted my focus towards applying machine learning to industrial process inspection, modeling, and control. In practical terms, my goal is to tackle high-impact problems by developing innovative computer vision and deep learning systems.
News
We've released EvoTorch, an open-source evolutionary computation library that enables effortless scaling on CPU/GPU clusters.
Our paper that devises ClipUp, a powerful optimizer for distribution-based policy evolution will also appear at PPSN XVI!
Our paper describing Neuromemetic Evolutionary Optimization, a novel way of mapping a search process to a continuous space using recurrent neural networks will appear at PPSN XVI.
Our paper on rephrasing program synthesis as latent continuous optimization got accepted to GECCO 2020!